Zero Tolerance AI… A Fictional (yet Cautionary tale)
Imagine this. A school district rolls out its shiny new AI-powered disciplinary tool, “Zero Tolerance AI.”
The promise? A safer, more equitable learning environment.
The pitch? Using a network of cameras and advanced AI image analysis, the system monitors student behavior, flagging potential issues like fights, bullying, or disruptions.
On paper, it’s an upgrade from relying solely on teacher referrals, which can be subjective. But soon, a disturbing trend surfaces: Black students are disproportionately flagged, even for minor actions like chatting in the hallway or wearing non-compliant attire.
The issue? Bias baked into the system.
Zero Tolerance AI (again… fictional, but stay with me) was trained on historical datasets—data shaped by decades of unequal disciplinary practices and skewed decision-making. What’s worse, its video analysis algorithms misinterpret cultural nuances, further reinforcing stereotypes. The result? Black youth are unfairly over-surveilled and over-disciplined.
If that’s not troubling enough, the constant surveillance raises another issue: privacy. Students are not just navigating a school system but are now under unblinking, algorithmic scrutiny.
This fictional scenario isn’t just a cautionary tale; it’s a real risk in how AI may be applied within educational settings. To understand how this happens, let’s break it down into five essential points about bias in AI.
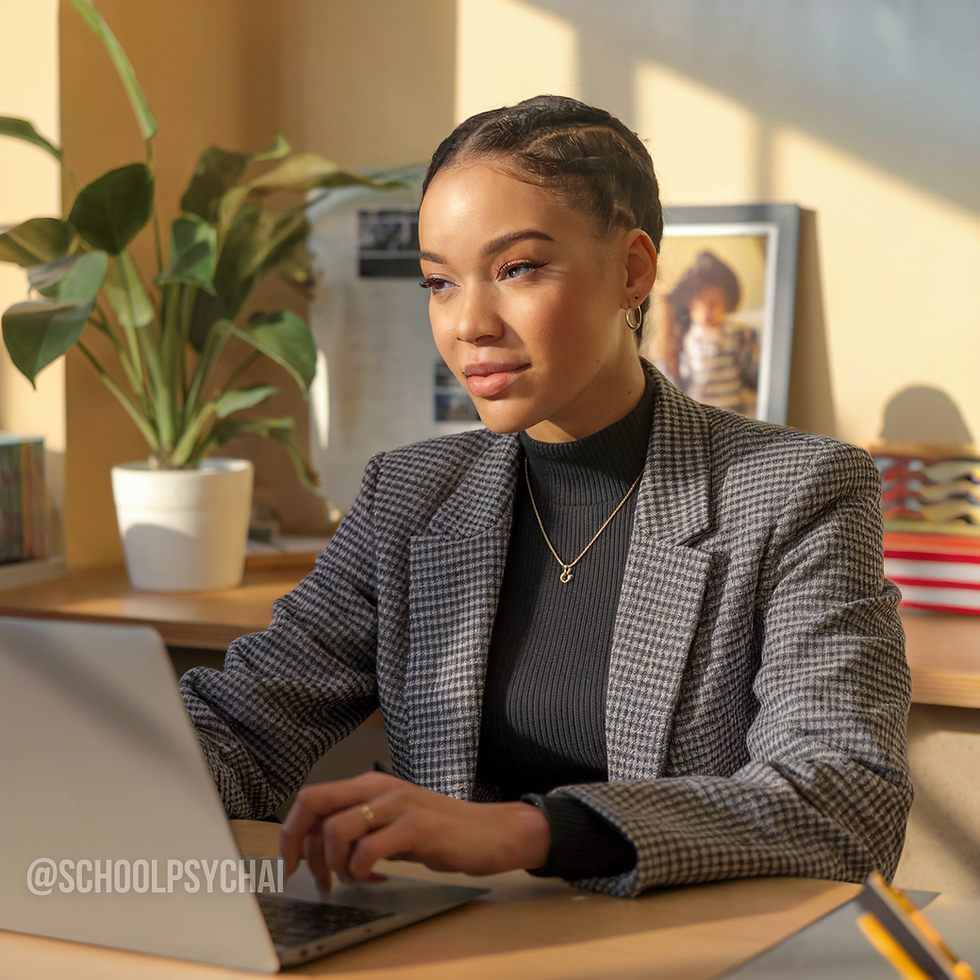
The Origins of Bias in AI
AI bias doesn’t appear out of thin air. It reflects the data it learns from and the algorithms it runs on. Think of AI as an overeager student. If you hand it a textbook full of inaccuracies, it will learn flawed lessons.
In Zero Tolerance AI’s case, the system learned from historical school disciplinary data. This data included decades of over-policing Black students, labeling them as “troublemakers” for minor infractions.
The AI absorbed these patterns and began associating Black students with higher disciplinary risks. Add poorly designed algorithms, and the bias gets hardwired into every system decision.
Types of Data Bias
Selection Bias: The dataset doesn’t represent the full population. For instance, disciplinary data might over-represent Black students due to historical inequities.
Coverage Bias: Certain groups are overrepresented. If most “examples of misbehavior” in training data come from a specific demographic, the AI will over-identify that group as problematic.
Historical Bias: When past injustices (like disproportionate disciplinary actions against Black students) are part of the training data, AI repeats them. The algorithms themselves are not neutral. They reflect the priorities of the developers. For example, if the developers weigh "verbal disruptions" heavily, cultural expressions like loud conversations or animated gestures could be flagged, disproportionately impacting certain groups.
Human Decision-Making and Historical Bias
Decisions made by teachers and administrators over the years were influenced by their own biases—conscious or unconscious. These biased decisions were recorded in disciplinary logs, creating a dataset that reflects and perpetuates those prejudices. When AI systems like Zero Tolerance AI are trained on this historical data, they inherit and amplify these biases, mistaking them for objective patterns.
Moreover, the integration of video surveillance adds another layer. Teachers, already influenced by biased historical data, may interpret behaviors through a skewed lens, further embedding bias into the system.
Let me make it plain.
We are talking about a vicious cycle that will only be amplified if we aren’t careful: biased human decisions lead to biased data, which in turn trains biased AI, resulting in more biased decisions.

2. Common Types of Bias in AI
AI bias wears many hats. Here are a few types most relevant to systems like Zero Tolerance AI:
Group Attribution Bias: Treating entire groups as though they share the same characteristics. In Zero Tolerance AI, this might look like associating Black students with “high risk” because the training data says so.
Implicit Bias: These are the unconscious assumptions coded into the system. The AI might unintentionally flag behaviors associated with specific cultural norms as disruptive.
Confirmation Bias: The AI reinforces patterns it “sees” in the data, even if those patterns are skewed.
What makes this particularly dangerous is that these biases aren’t easy to spot. They operate quietly, influencing decisions that can have serious consequences for students.
3. The Consequences of AI Bias
When bias creeps into AI, the fallout isn’t theoretical—it’s personal and societal.
Discrimination: Black students face harsher punishments, perpetuating cycles of disadvantage. It’s not just unfair; it’s damaging. A single suspension can derail a student’s academic trajectory.
Erosion of Trust: When parents and communities see that an AI tool unfairly targets their children, trust in the school system crumbles.
Privacy Concerns: Tools like Zero Tolerance AI aren’t just biased; they’re invasive. The idea of students being watched 24/7 by cameras analyzing their every move feels dystopian.
And let’s not forget the legal and ethical implications. Schools risk lawsuits and public backlash when biased systems are exposed.
4. How to Mitigate AI Bias
Fixing bias isn’t easy, but it’s possible with intentional effort:
Start with Better Data: Training data must reflect the diversity of the population. For schools, this means ensuring datasets represent students equitably across all demographics and behaviors.
Audit Algorithms: Regular audits can reveal how different groups are impacted. Are Black students being flagged more often? Why? Audits shine a light on these disparities.
Transparency Matters: Schools using AI must make it clear how these tools work. That includes explaining how decisions are made and providing avenues for oversight.
Human Oversight: AI doesn’t replace humans—it assists them. Educators and counselors must review AI-generated flags to ensure fairness and context are considered.
5. Organizations Tackling AI Bias
Addressing AI bias isn’t a solo mission. Several organizations and initiatives are working to ensure AI is developed and used responsibly:
School Psych AI: Dedicated to supporting school psychologists, School Psych AI prioritizes ethical AI practices. The platform focuses on fairness, transparency, and cultural sensitivity in its AI systems. For example, when developing its redacted model, School Psych AI used diverse datasets to ensure a wide range of names and backgrounds were represented. This careful approach helps reduce workloads for psychologists while ensuring that all students are treated equitably.
The Algorithmic Justice League: Advocates for equitable AI systems and raises awareness about algorithmic bias. This organization combines research, storytelling, and activism to hold AI developers accountable.
Weapons of Math Destruction: While not an organization, this book by Cathy O’Neil, was recommended to me a little over a year ago. It was an easy read and highlights the dangers of algorithmic systems when they are applied without proper oversight. It serves as a wake-up call for developers, policymakers, and the public to recognize and combat bias in AI.
The Partnership on AI: This multi-stakeholder group unites tech companies, academics, and civil society groups to develop standards and best practices for AI ethics. They provide frameworks for organizations to address bias effectively.
These initiatives and resources offer valuable insights and tools for building fair and equitable AI systems. Whether through direct action, advocacy, or education, they help ensure that AI serves all communities without perpetuating harm and reducing bias.
Conclusion
Bias in AI isn’t a technical glitch—it’s a human problem, mirrored and magnified by technology.
The potential of AI to improve fairness and equity, as explored through the hypothetical "Zero Tolerance" example, hinges on deliberate design and responsible use. Unless we actively guard against treating AI as inherently unbiased, we risk amplifying the very inequities we aim to address.
By learning from stories like this, we can build a future where AI serves all people equally. It starts with understanding, accountability, and a commitment to fairness. The stakes are too high to get this wrong—especially when the next generation is watching.
Written by Dr. Byron M. McClure. Follow School Psych AI across social media platforms including Facebook, YouTube, Tik Tok, Instagram, and LinkedIn. Check out our website to learn more about School Psych AI. Works Cited
Comments